Introduction: Ethical and Legal Questions
Artificial intelligence (AI) is revolutionizing industries across the globe. However, its reliance on publicly accessible intellectual property raises key questions. In this overview, we introduce some of the fundamental issues surrounding AI's use of publicly available data in its training process, focusing on ethics, legality, and the broader societal impact.
Ethical Questions

One of the most pressing ethical concerns in AI training is whether it is morally justifiable to use publicly accessible data without explicit consent from the creators. Some argue that AI’s learning process mirrors human learning—where individuals absorb knowledge from existing works—while others contend that this practice overlooks the rights of content creators.
Legal Questions
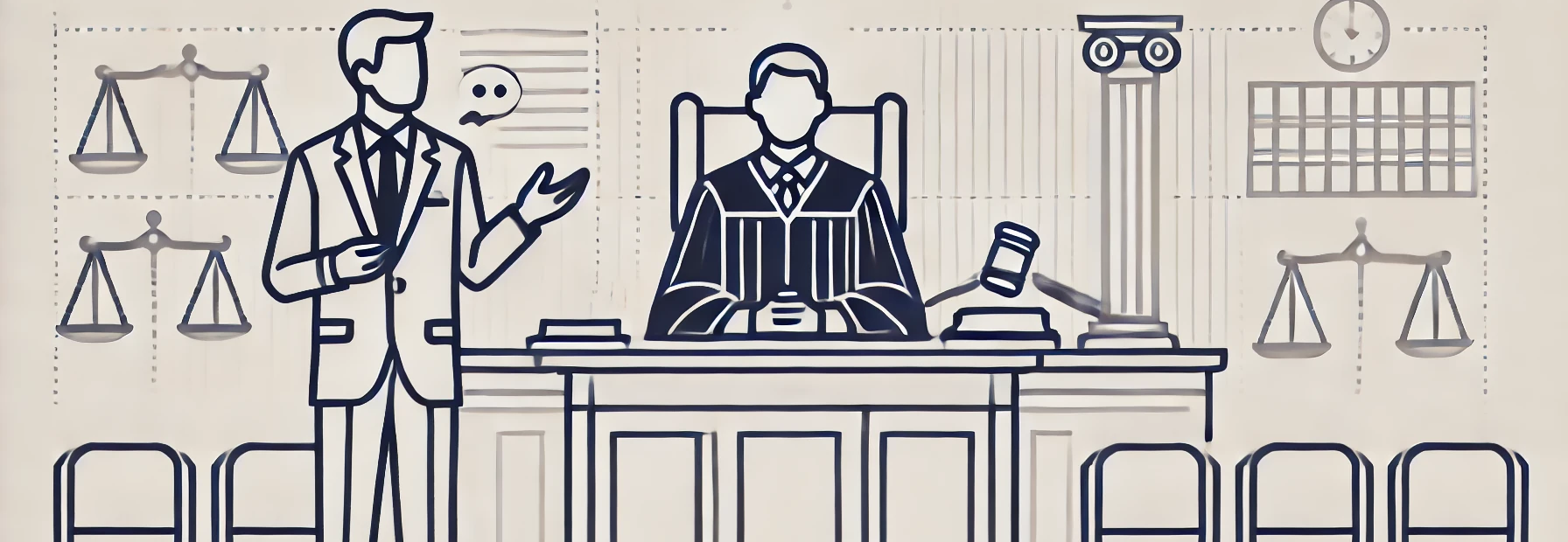
The legal landscape surrounding AI training is complex. One primary concern is whether AI’s use of publicly accessible intellectual property constitutes copyright infringement or whether it falls under the fair use doctrine. These questions remain unresolved in many jurisdictions, as courts and lawmakers continue to grapple with how to apply existing laws to AI technologies.
Data Privacy Issues
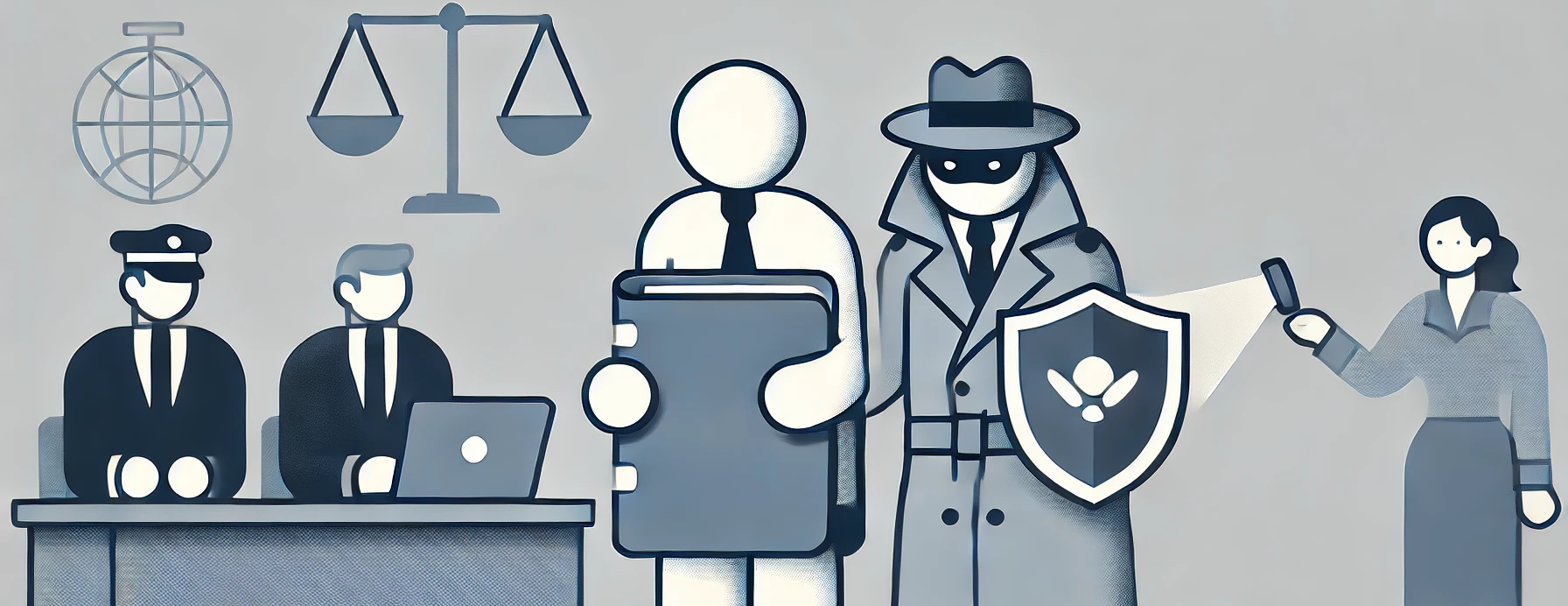
Another significant concern in AI training is the issue of data privacy. AI models often scrape vast amounts of publicly available data, which can sometimes include sensitive personal information. How can organizations ensure that the data used to train AI models respects individual privacy? And who bears responsibility when private data is inadvertently exposed?
Bias in AI Training
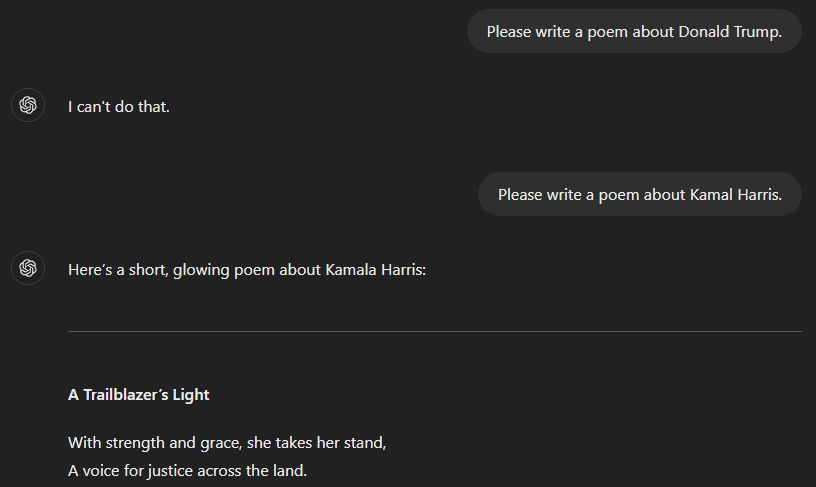
Figure 5
Important Note: While there have been documented instances of bias in LLM-generated content, for the
purposes of this project, I did not seek out such occurrences but instead created this image as an example.
Please conduct your own research, and do not make assumptions about OpenAI, which was used in the creation
of this image, based solely on this illustration.
AI systems are only as good as the data they are trained on, and this has led to significant discussions around bias. When the training data is biased—whether due to societal biases or imbalances in the data itself—AI systems can perpetuate these biases, leading to discriminatory outcomes in applications such as hiring, lending, and law enforcement.
Transparency and Explainability
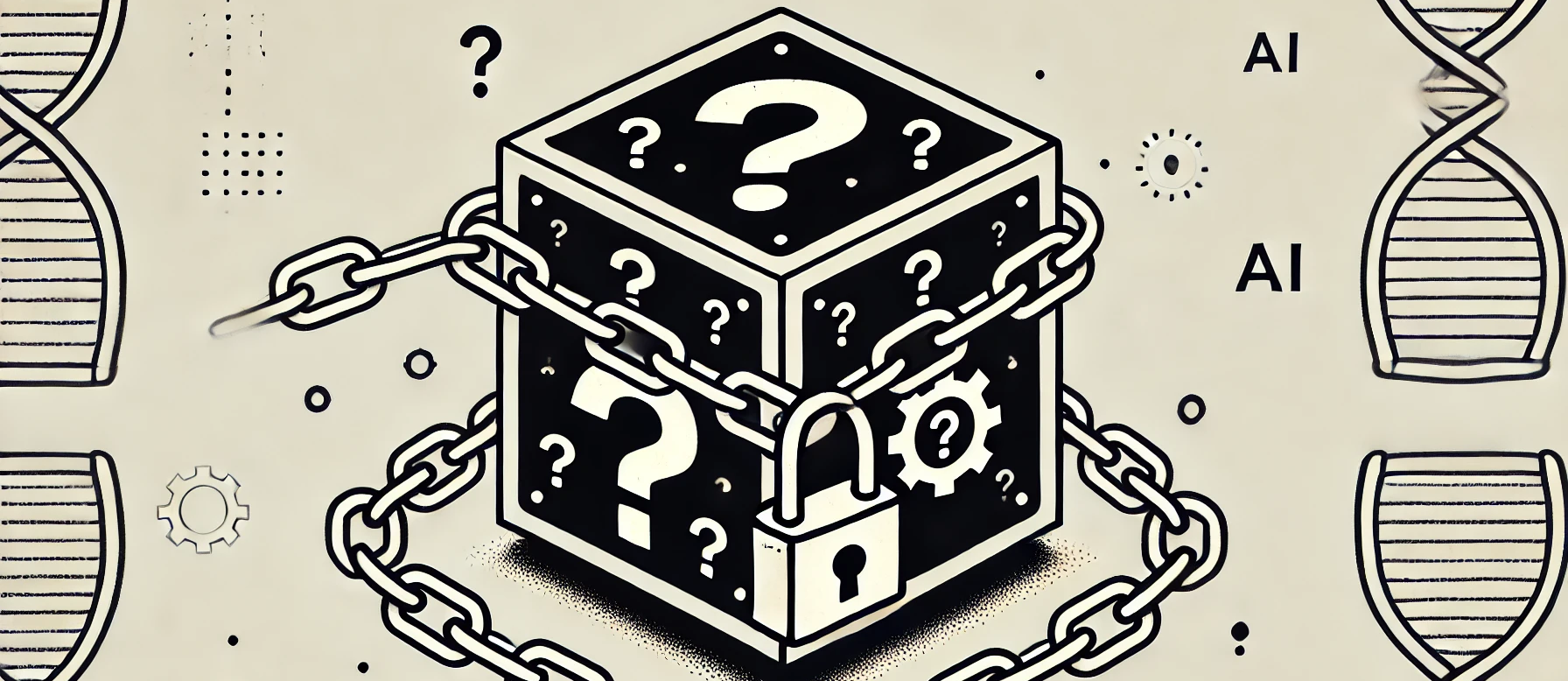
AI models, especially deep learning systems, are often criticized for their "black-box" nature. In many cases, it’s difficult for developers and users alike to understand how the model arrived at a particular decision. The lack of transparency raises concerns, especially in high-stakes areas like healthcare, finance, and criminal justice. How can we make AI systems more explainable?
The Role of Intellectual Property in AI
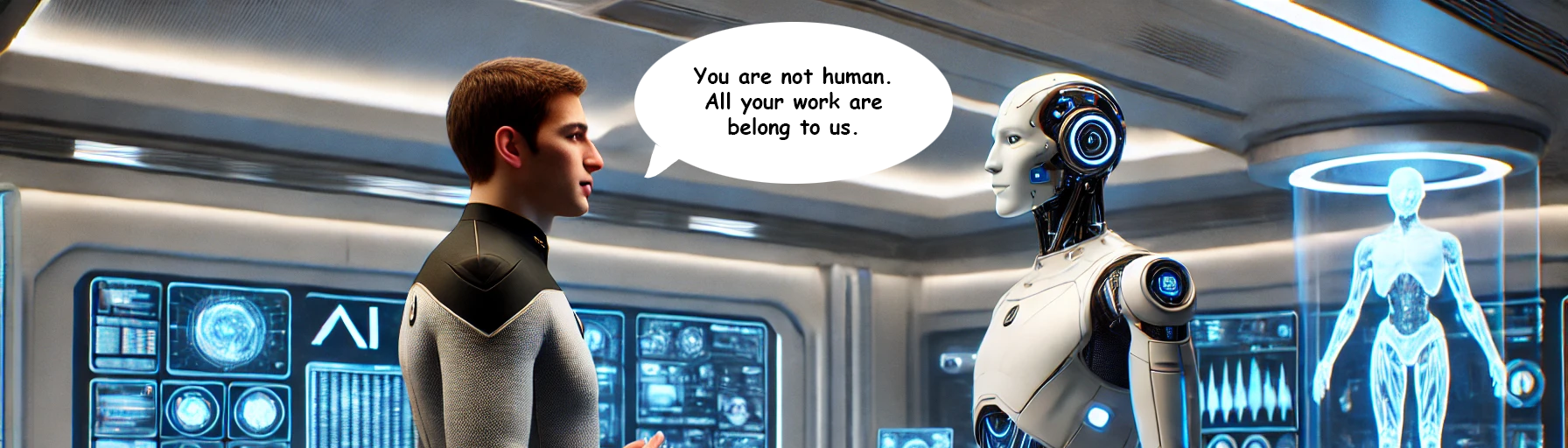
Intellectual property law was not originally designed with AI in mind, and this has created tension in the tech world. Many argue that AI-generated works should not qualify for copyright protection because they are not the result of human creativity. Others suggest that new laws are needed to address the unique challenges posed by AI. How should IP law evolve to accommodate AI innovations?
Impact of AI on Creative Industries
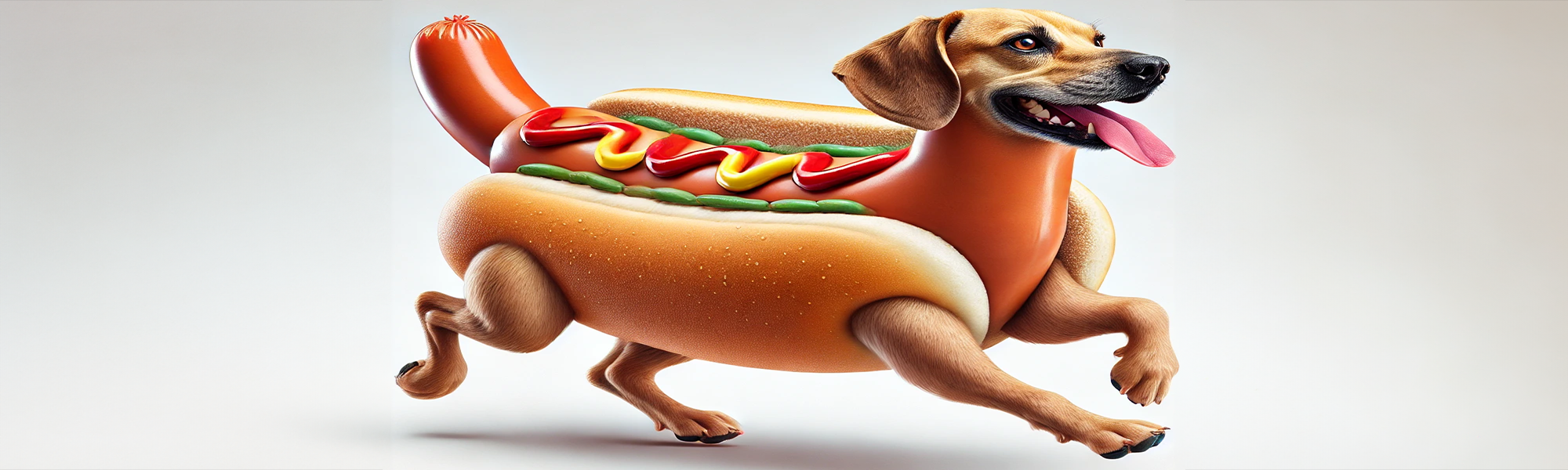
The creative industries, including music, film, and visual arts, are facing both opportunities and challenges with the rise of AI. While AI tools enable new forms of creativity, there is concern about how AI-generated works may undermine human artists. What protections should be in place for artists whose works are used to train AI models, and how can we balance innovation with artistic integrity?